Prediction of protein SUMOylation sites and SUMO-interaction motifs by deep learning.
1. Small ubiquitin-like modifiers (SUMOs) play an essential role in the regulation of a variety of biological processes.
2. SUMOs could modify proteins by conjugating with specific lysine residues or by non-covalently interacting with motifs.
3. Traditional shallow machine learning methods failed to learn the underlying biological features of protein SUMOylation sites and SUMO-interaction motifs.
4. In this work, we established a predictor called DeepSUMO using deep learning methods for predicting SUMOylation sites and SUMO-interaction motifs.
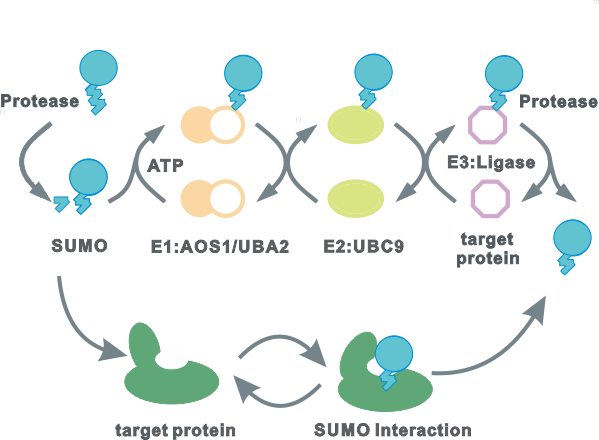
Robust deep learning model of DeepSUMO
1. Concatenated convolutional neural networks are utilized for pattern extraction from three coding methods, one-hot coding, modified PSSM and KNN coding.
2. Our robust deeplearning model is implemented with mini-batch stochastic gradient descent, L2 regularization and rectified linear unit activation.
3. DeepSUMO outperforms other prediction tools and also the traditional machine learning algorithms.
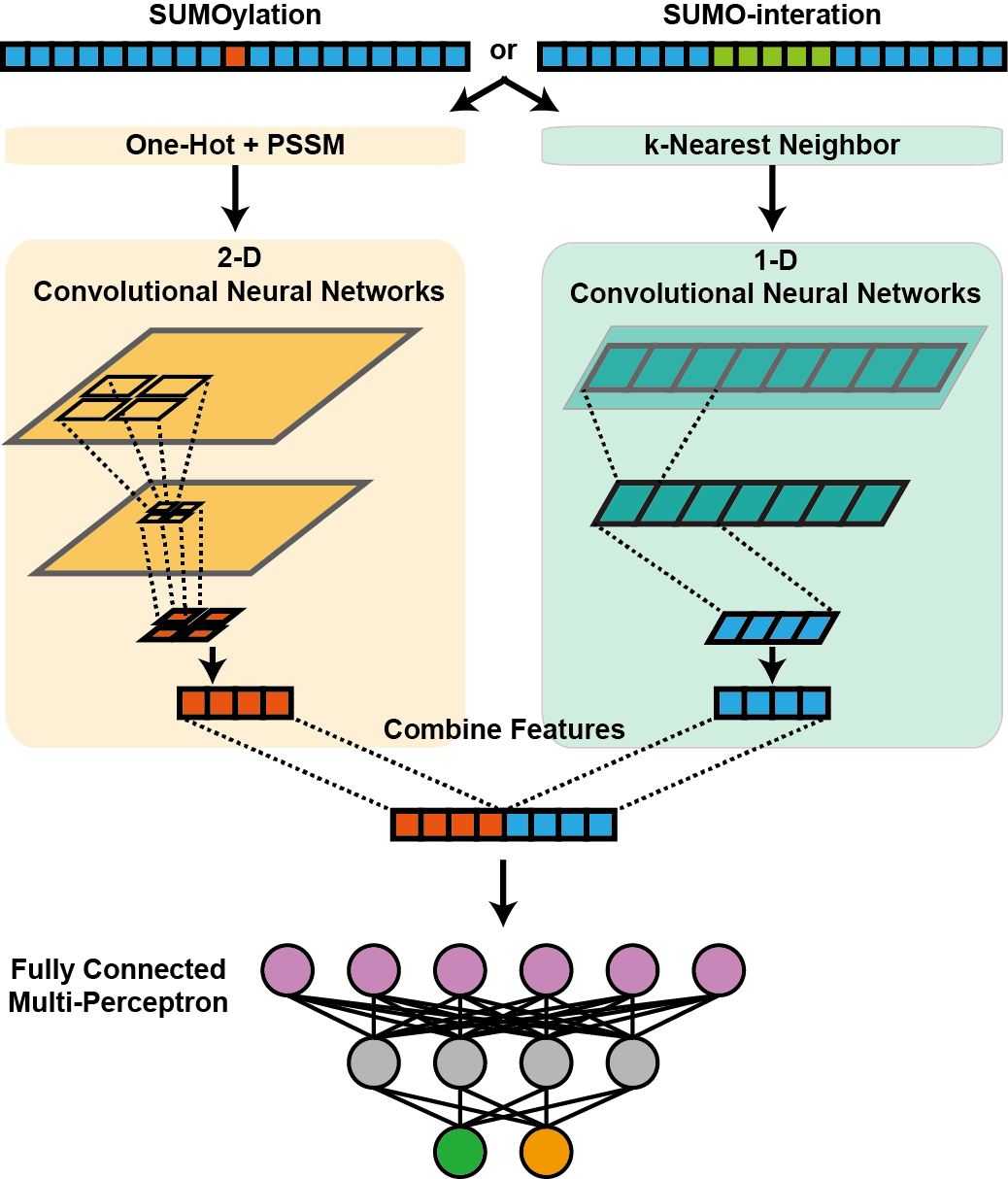
1. Concatenated convolutional neural networks are utilized for pattern extraction from three coding methods, one-hot coding, modified PSSM and KNN coding.
2. Our robust deeplearning model is implemented with mini-batch stochastic gradient descent, L2 regularization and rectified linear unit activation.
3. DeepSUMO outperforms other prediction tools and also the traditional machine learning algorithms.
Visualization
1. To assist further analysis, DeepSUMO implements an automatic pipeline for visualizing the prediction results.
2. DeepSUMO can present the graphical representation of the inputted proteins together with their predicted resultsand domain organization in the visualization panel.
1. To assist further analysis, DeepSUMO implements an automatic pipeline for visualizing the prediction results.
2. DeepSUMO can present the graphical representation of the inputted proteins together with their predicted resultsand domain organization in the visualization panel.
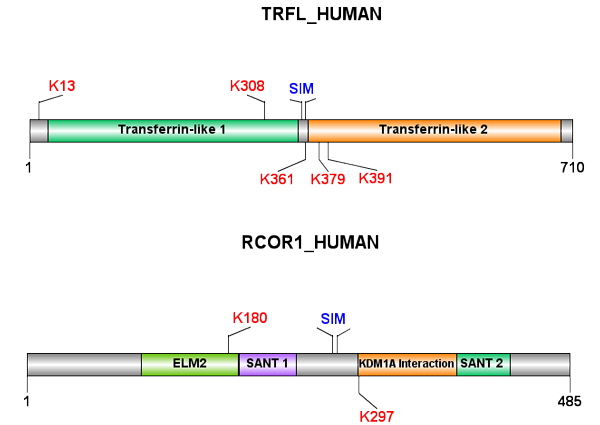